- News and Stories
- Blog post
- Principles & Practices
Delivery-Driven Policy
Why is policy still educated guesswork with a feedback loop measured in years?
At Code for America, we envision a government that works for people, and by people, in a digital age. The framework of delivery-driven government is intended to give a more concrete picture of that vision, and describe the ways that government must begin to operate in order to achieve it. In our original paper defining delivery-driven government, we said:
The movement to modernize government technology has been focused on the delivery of government services using modern technology and best practices. But that is only half the solution; now we must also learn to drive policy and operations around delivery and users, and complete the feedback circuit. Only then can we effectively achieve the goals government policies intend.
This is the first in a series of papers about delivery-driven policy, which builds on the overarching concept of delivery-driven government with a focus on policymaking and advocacy. In it, we explain the key disconnect between policymakers and government delivery, introduce delivery-driven policy as a concept, and offer examples of delivery-driven policymaking—informed both by our own work and that of others in the field. In collaboration with New America and the Delivering Better Outcomes Working Group hosted by the Beeck Center for Social Impact and Innovation at Georgetown University, with whom we’ve developed these ideas, we invite all readers to share their own examples of delivery-driven policy at resources@codeforamerica.org. In subsequent papers, we will go deeper into some of the examples introduced here, highlight new examples submitted by our wider community, and expand on these ideas as they relate to adjacent fields—including advocacy, procurement, and digital talent in government. You’ll find these on the delivery-driven government page of Code for America’s website.
The problem: an implementation gap
Policymaking is in a quiet crisis. While there are meaningful differences of opinion in the United States and elsewhere about which policies will result in the kind of society we want (often driven by different visions for our society), it’s also true that many of the policies that result from our democratic processes simply do not live up to their intent. It’s like a car where the steering wheel is only loosely connected to the wheels: We might fight over who is in the driver’s seat, but regardless of who is driving, you’re not going to get where you meant to go—and you’re going to hurt people along the way.
Why is there such a profound disconnect? To be clear, implementation is often derailed from its intent by the deliberate interference of people who oppose the policy. But much of the failure is not deliberate; it is the result, in part, of an outdated model that keeps policymaking and policy implementation as separate domains, with separate skills and incentives. The impact of these outdated models becomes increasingly dire in a shifting landscape where the work of government grows more and more complex.
How might the shift to a digital world affect government’s ability to implement policy? In theory, government could use digital technology to build programs with the feedback loops needed to course-correct over time, effectively steering the car. Some have suggested we just need to invest more in technology for government; but it’s not how much government spends, it’s how government spends it. Constrained by antiquated procurement practices, and focused on outputs rather than outcomes, the US spends $200 billion a year on digital technology but frequently lacks the data and insights needed to effectively manage the programs it funds. And it is particularly hard when measuring those outcomes requires crossing silos, as our government was designed around discrete functions in a pre-internet era.
But Code for America, the United States Digital Service, 18F, and many others have made the case that a user-centered, iterative, and data-driven approach can result in digital technology that provides those much needed data and insights, and at a far lower cost. The real benefit, however, is when those same practices—user-centered, iterative, and data-driven—are applied to the policymaking process as well.
What is delivery-driven policy?
Today, emerging models of policymaking and delivery, both theorized and increasingly practiced, are resulting in better fidelity to the intentions—reconnecting the steering wheel to the wheels of the car. These models involve integrating policymaking more tightly with its implementation, and iterating on both in consistent cycles. They serve in stark contrast with traditional “waterfall” models, in which policy development is a distinct project phase managed by a distinct team with distinct skills, and implementation begins with a separate team with separate skills after policy is set. The traditional model looks something like this (borrowed from former Code for America Product Manager Jake Solomon’s 2015 talk at Code for America Summit):
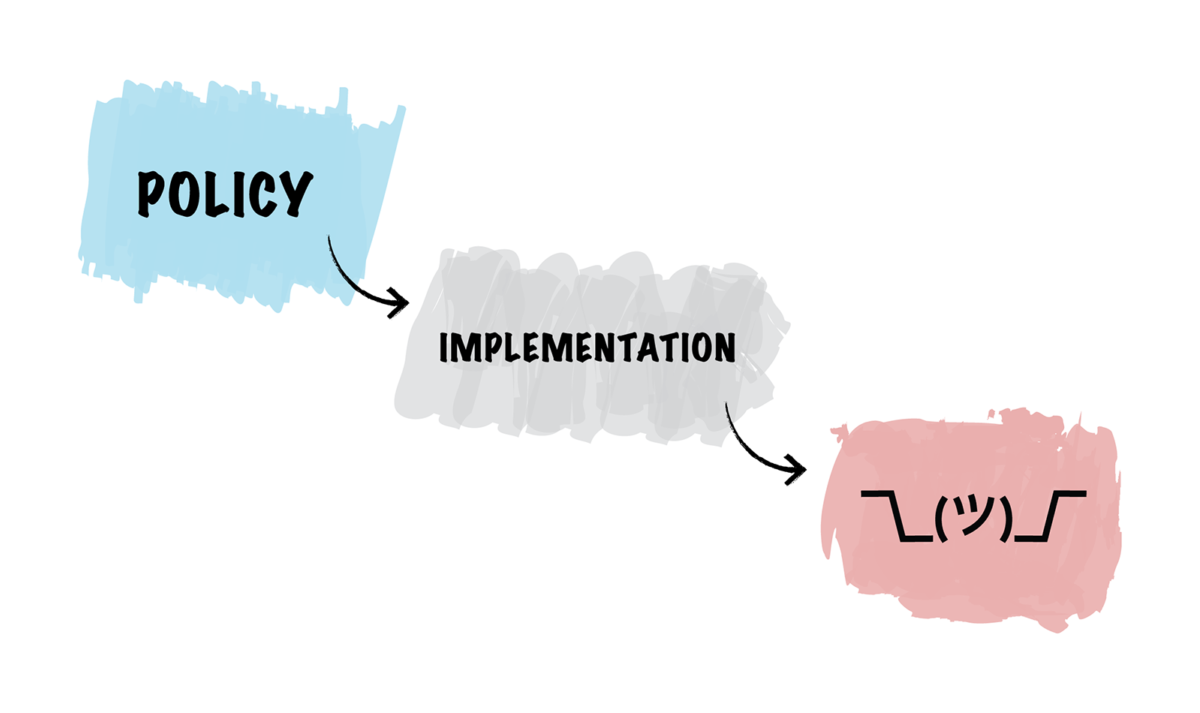
By contrast, a delivery-driven policy process assumes policymakers will get things wrong the first time. It seeks to be agile, iterative, and user-centered, with how the policy will be delivered informing its development from the start. This requires multi-disciplinary teams that include digital and design professionals, with the skills to accurately understand and solve for user needs, alongside policymakers, subject matter experts, and other stakeholders like procurement and compliance professionals.
In this model, teams start small and iterate progressively on both the policy and delivery together. They also build instrumented delivery systems that provide near-real-time feedback, creating mechanisms for experimentation that can inform policy development with the knowledge of what’s actually working towards the original intent.
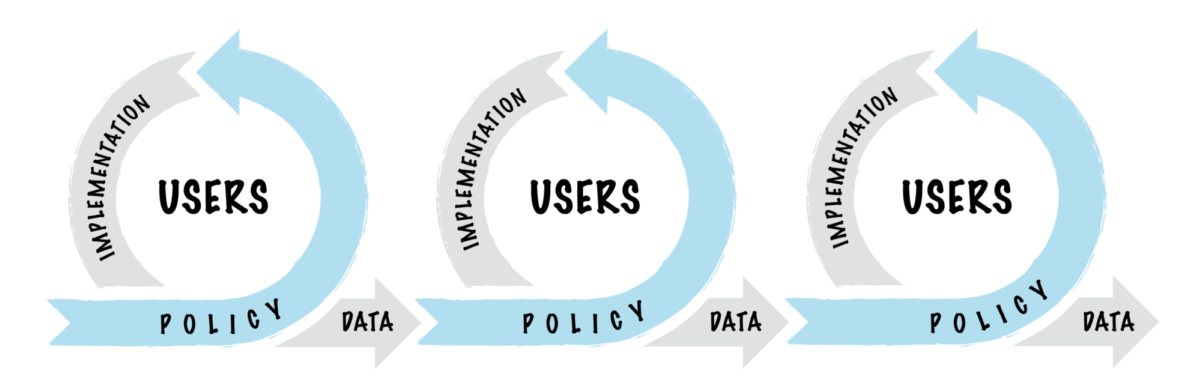
How waterfall-style policy leads to an implementation gap
While implementation gaps are visible everywhere that policy (especially high-level policy) fails to live up to its intent, we want to start to give specific, concrete examples of lower-level policy failures where the mechanisms of failure are easy to see—at least in retrospect. An example from our work at Code for America on closing the participation gap in the Supplemental Nutrition Assistance Program (SNAP) in California illustrates the dangers of the traditional “waterfall” approach described above.
Federal regulations require that if you want SNAP benefits (colloquially referred to as food stamps), you must complete an interview with an eligibility office. In California and many other states, the standard practice had been for eligibility offices to schedule interviews without consulting the applicant—which means they were often set for times when applicants are at work, in class, or otherwise unable to take the call. Realizing that this was keeping eligible people off SNAP, the USDA created a “modernization policy waiver” that gave states the option to offer applicants “on-demand” interviews whenever it was convenient for them to call during working hours.
The problem was that the waiver created a very specific definition for the term “on-demand interview,” with strict parameters in how it could be implemented. One of those strict parameters specified switching the entire state or county from pre-scheduled to on-demand interviews all at once, because there’s a principle in state and federal regulations about treating every client the same. In the interest of fairness, federal regulators made experimentation and phased-in implementation impossDownload the full paperible within the context of this waiver.
Almost a decade later, only nine states conduct on-demand interviews. California is one state that was highly incentivized to take advantage of this; it had one of the lowest participation rates in the country and a generally pro-welfare political environment. With 40 million people and high rates of poverty, it’s also the state that would have moved the needle on access the most by streamlining its enrollment process. California actually applied for and received the on-demand interview waiver in 2013, with a two-year window for implementation. The state told the counties, who administer the program, that they could opt in to use the waiver, but no county felt they could take the risk of an overnight switch to a new system. California lost the waiver in 2015 without ever using it. We believe this may have been a barrier in other states as well, especially other county-administered states.
How could this have been avoided? First, waiver language could have simply been less specific. States and counties had long had the option to implement many different types of flexible, client-initiated interviews without a waiver or formal approval, and they were doing this in many states. The new overly-precise definition of “on-demand” created the perception that there was only one way, and suddenly those options for flexibility appeared off-limits at the same time that the new official way to conduct client-initiated interviews was untenable. We are certainly not the first critics of policy to point out that over-specifying tends to have negative consequences. Sometimes those consequences are in fact the exact opposite of what the policy intended.
If the USDA had been in better communication with eligibility offices while drafting the policy, user-testing their assumptions, they might not have written the waiver language with an all-at-once restriction in the first place. They also could have made some estimates about how many and which states they hoped would try the waiver in a given time frame, and if the number was lower than they hoped, they could have looked further into what was holding them back. And to be fair, because we don’t really know the USDA’s story, it’s possible they did this, but encountered their own barriers to adjusting the policy. Regardless, the policy waiver still stands as originally written; and, at least in California, rather than encouraging eligibility offices to innovate on behalf of applicants, it stopped innovation. Because the policy was not user-centered, iterative, or data-driven, millions of applicants continue to be put through a burdensome and ineffective enrollment process at a time in their lives when they most need support.
It’s easy to look back at this story as one of policy that failed to live up to its intent, and it’s often helpful to analyze policy interventions after the fact—especially when policymakers are considering options and want to know what’s been successful elsewhere, as practitioners of evidence-based policy do. Our goal here is to further the notion that policy doesn’t need to be a static set of rules, but can be guided towards better outcomes as it is being implemented, if both policy and implementation are done in a collaborative and iterative fashion. It’s a bit like the difference between using data as a compass versus data as a grade: A compass helps steer you in the right direction during your journey, while a grade is an after-the-fact assessment of success that you can’t do much about now that the work is done. In this regard, delivery-driven policy is a natural extension of and complement to the field of evidence-based policy.
Use data as a compass, not a grade.
That’s why delivery-driven policy is tied in many cases to modern technology systems—or hacks to those systems—to allow for instrumentation, real-time (or near-real-time) situational awareness of the impact of operations and policies on users, and continuous improvement. The delivery of most consumer services today is highly instrumented and gives a wide variety of actors within the system the means by which to steer. With all due consideration towards privacy and ethics consequences, we need to become competent at instrumenting and constantly improving the delivery of the services that matter most in our society: our public services.
Through our work at Code for America and in the work of others, we have participated in and observed examples of delivery-driven policymaking in practice. We’ll highlight a few examples below, but again, we encourage submissions from the community at resources@codeforamerica.org.
Example 1: Medicare Regulators and Real-Time User Testing
“I’m realizing that what I’m holding is 500 pages of untested assumptions!”
When federal regulators are charged with implementing a law, common practice is for the regulators to spend months detailing the rules that will govern implementation. Often, a tech team (usually an outside vendor) will be charged with encoding those rules in the form of a website that users will interact with. Traditional development methods call for “user acceptance testing” at the end of the project, which is generally too late for meaningful feedback. Agile development, which insists on user feedback throughout the process, is becoming more accepted, increasing the quality and decreasing the cost of projects in the public sector. But while the tech teams may be able to learn from users, they can be very constrained in how to respond by the parameters set out by the regulators, who rarely see how what they’ve written plays out in the real world.
In 2016, regulators at the US Department of Health and Human Services (HHS) were charged with implementing the Medicare Access and CHIP Reauthorization Act of 2015 (MACRA), which was meant to enable Medicare to incentivize better care via changes to the payment system for physicians who treat Medicare patients. HHS tapped a team from the United States Digital Service led by Mina Hsiang. Mina and her team proposed to do things differently.
Instead of waiting months for the final regulations to be delivered, Mina asked for a first draft and had her team build early prototypes of website features based on the draft. They tested these prototypes with real users, and shared data and feedback with the regulators. In testing a “setup wizard” assistant for doctors, for instance, they found that the criteria for enrollment were not actually mutually exclusive and collectively exhaustive (see the MECE principle), which meant that doctors could fall into multiple buckets. This was not only extremely confusing for doctors trying to use the service, but it led to users who were not properly enrolled. This problem had not been visible to the policy team, but because Mina’s team was able to surface it in an early implementation, they were able to engage the policymakers in changing in the language of the rule (and the tools), and also changing the criteria (when the rule was finalized).
Needless to say, in a traditional policy and delivery team relationship that did not involve real user feedback during the building of the service, these problems would have become apparent when the service was already operating at scale, compounding problems for doctors and administrators alike. It’s also likely that the vendor, having delivered to the specification they’d been handed, would have been moved into a maintenance contract, making it hard to quickly make the changes that were needed. In a waterfall approach, delivering on the specification is the end of the work. In an agile, delivery-driven approach, it is the beginning of figuring out how to make the service really work for its users.
Another way the delivery and policy teams worked together was around how doctors received data and information about their performance. Remember, the point of MACRA was to incentivize doctors to provide better care for Medicare patients by paying them more when patients reported better outcomes and better experiences, when care was better coordinated, and when services were used appropriately, according to the measures the program designed. This meant that it was critical that doctors understood when and how often they’d met those criteria, so the system was supposed to collect data from doctors and provide analysis back to them.
In this case, Mina’s team looked at how doctors used the existing services from the programs that MACRA was intended to replace. They found that doctors were asked to upload their data in ways they found enormously confusing (and were often left wondering if the submission had even gone through) and then a year later they were invited to download a PDF report containing a series of charts which they didn’t really find useful. These previous systems had been built at enormous cost, for the exact purpose of providing this analysis to doctors, but only about 5% of doctors were even using it. (If you divide the cost of the feature by the number of doctors pulling their reports, CMS was spending about $1M per report.) Armed with this insight, Mina’s team was able to work with policymakers not just to improve on the upload features and change the reports, but to change the process by which they would be designed. Policymakers decided to hold off on defining what the report should contain in the final rule, and Mina’s team instead helped them outline a user research-driven process that would take place later in the year which would iteratively determine what data doctors could get back and how it would be presented. The result was a report that doctors actually use.
Even the name of the program (not generally the domain of the tech team) benefitted from user testing. Many of the proposed names were confusing to the doctors, but it was important that this sounded like something they could understand and trust. The delivery team took just two days to talk to 50 doctors using a questionnaire and brought the data back to the team. This research led to calling it the Quality Payment Program (QPP).
The website and the regulations went through many more iterations before the QPP team called the rules final. The results of these collaborations were happy policymakers, happy doctors, and a program that is succeeding in its goals. Because policymakers could see how users experienced and interpreted the rules they’d drafted, and make changes to make them work better, they were proud of their work. Many of them reported that they’d just written the best rules of their career, and Medicare patients are now getting better care.
Example 2: Implementation as Policy in RAP Sheet Fee Waivers
The above example is an excellent illustration of delivery-driven policy as fundamentally user-centered, iterative, and data-driven. This next example speaks more to a direct relationship between delivery and policy. Lawrence Lessig has said “code is law.” In this case, a bit of code became policy.
In California, one of the first steps in clearing a criminal record is getting a copy of your Records of Arrests and Prosecutions, or RAP sheet. There’s a $25 fee to obtain your RAP sheet, but that fee is technically waived for people with low incomes. However, until recently, applicants had to request a special form, wait for it to arrive in the mail, fill it out, return it in the mail, wait again for another form to come back, and finally return to the office with the new form to get the fee waived. People with low incomes had two choices: Pay the fee anyway, or wait at least two weeks longer than others to complete this step.
In 2017, a Code for America Fellowship team worked with the California Department of Justice (DOJ) to put the form online, saving low-income applicants many days of delay in a process that can be very urgent for them, especially if they’re trying to pass a background check for a job. But when the team put the form online, they also realized they could solve another problem: The DOJ was using a single, flat cutoff—the federal poverty line—to calculate eligibility, regardless of where residents lived. That meant residents in high cost-of-living counties (like San Francisco or Los Angeles) were being denied waivers even if they had a low income by local standards.
The team realized that the DOJ was not opposed to using a more localized eligibility calculation, but because they had been receiving the data from applicants on paper, they had to manually calculate the person’s eligibility, which includes household size as well as income and county. A more accurate calculation of eligibility would rely on the Department of Housing and Urban Development (HUD)’s county-by-county definitions of poverty. Through conversations with the DOJ, the Fellows realized the DOJ staff had limited capacity and a high workload, which influenced them to rely on an overly simplistic, manual eligibility calculation process.
Make the right thing to do the easy thing.
But in putting the waiver form online, the Code for America team was able to add a drop-down menu so applicants could select their county. The online form would then instantly look up that county’s poverty cutoffs for various household sizes according to HUD data. From a technical perspective (with all due respect to our team!) it was not difficult to program the form to access the data and to do these calculations automatically. With these small changes, our team effectively changed this policy, and made the process not only more accessible, but also more equitable.
We told a friend of Code for America who works in criminal justice reform advocacy about this project. She was astonished. “We can work for years to get a jurisdiction to agree to something like that!” was her response. “How did you get them to agree to it?” The truth is, we didn’t. Or at least, we didn’t start by trying to convince them of the need for a policy change. We just did what former Code for America Head of Product Cyd Harrell has often said should be the goal of government technology: Make the right thing to do the easy thing.
Key lessons of delivery-driven policy
- Iterate on implementation from the start. Rather than writing policy and then handing it off to delivery teams, multi-disciplinary teams can work on policy and delivery in tandem. Integrating policy more tightly with implementation, and iterating on both in consistent cycles, will result in policy that can both be delivered effectively and live up to its original intention. As Cecilia Muñoz has frequently said, you need the digital delivery people at the table from the start.
- Build for instrumentation. Modern technology architectures and tools make it much easier to see how systems are performing, and how real people succeed and fail while interacting with them. Instrumented systems can provide near-real-time feedback and insights that can help both delivery and policy teams adjust both as needed.
- Have some digital capacity inside government. If the strategy and delivery of digital services is entirely outsourced, there is no one with sufficient power to reshape the waterfall process into a user-centered, iterative, and data-driven one. And if you have to pay a vendor a large fee for every change to your spec (because that’s the way traditional contracts are written), it’s very hard to be iterative. Having people inside government who are good at user-centered, iterative, and data-driven development helps, even if they still work with outside vendors, which is the norm.
- Delivery-driven policy does not necessitate more spending. As Clay Shirky said: “the waterfall method amounts to a pledge by all parties not to learn anything during the actual work.” Learning as you go should cost less in the long run as you avert larger, more expensive failures that aren’t seen until it’s too late.
Practicing delivery-driven policymaking means bringing user-centered, iterative, and data-driven practices to bear from the start and throughout, often through partnership with delivery teams. It means getting deep into the weeds of implementation in ways that the policy world has traditionally avoided, iterating both on policy and delivery. By tightly coupling policy and delivery, governments can use data about how people actually experience government services to narrow the implementation gap and help policies get the outcome they intend.
What’s next?
Public servants don’t make policy in a vacuum. A wide variety of advocates can be at the table, and bring their own assumptions about what policies will result in the outcomes they seek. They can also suffer from paying too little attention to the implementation gap, and end up caught in the trap of unintended consequences. Our next article will explore what delivery-driven advocacy could look like, and how bringing these practices to the advocacy community could benefit the people government serves. For a preview, check out Sarita Gupta’s talk from the last Code for America Summit, where she spoke about how Caring Across Generations wants to radically improve our country’s outdated care system by bringing insights from delivery to the policy discussion.
We will continue to share stories and thinking around delivery-driven policy and advocacy from both our own work and other examples we’ve come across. We will do this in partnership with New America’s Public Interest Technology program, Georgetown’s Beeck Center, and others. We welcome contributions and feedback to us at resources@codeforamerica.org and to our partners, and we encourage readers to learn more about delivery-driven government.