- News and Stories
- Blog post
- Principles & Practices
Five Questions to Ask Before Adopting AI in Government
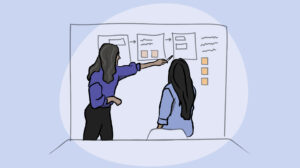
All across the country, government agencies at every level are considering how they might leverage AI. It’s a technology with the potential to make things easier for agencies handling a vast number of cases, as it can process huge amounts of information more efficiently than a human could alone. It can be useful in applications like automatic record clearance and helping sort client queries and applications for public benefits, along with a lot of other use cases.
But to be successful, AI needs to be deployed responsibly. Like any technology that’s new to government, AI presents a wealth of opportunities and challenges simultaneously. It may require previously siloed departments to coordinate and collaborate on rollout strategies. Teams might have to write new policies about data management and client privacy. Agencies will have to try pilot projects and measure their success, pivoting as needed based on the feedback they’re getting. Along the way, they’ll also need to explore new procurement challenges, working with vendors as they pilot and measure AI projects.
In short: Thinking through the implementation of AI in government is a big task. So here are five questions government teams should be thinking through when they want to experiment responsibly with AI.
Is the approach tailored to people’s needs, and can we measure its success?
“Should we be doing this?” may sound like an obvious first question, but it’s a critical one. Just because a technology is available doesn’t mean it’s the best fit for a given situation. Any application of AI should be tailored to the specific needs of the government staff who will be using it and the people who will be served by it. To understand if AI is the right fit, teams need to understand the pain points of a process, and can find this out by conducting qualitative and quantitative research—meaning analyzing data and doing interviews with caseworkers, administrators, and other government employees, and with the people using the services they provide.
Then to understand if AI has been successfully deployed to address those pain points, there needs to be a plan to measure its value. Clearly defining metrics at the beginning of a project—both those that would define a stopping condition and that would indicate success—means AI experimentation can be done more confidently. This kind of measurement ensures that the people who are impacted are placed front and center when considering next steps.
Will this application of AI improve equity?
Put more plainly: Will the benefits of using AI be felt by everyone? If not, who is likely to benefit—and who isn’t? AI gets trained on data, and automating processes introduces some risk that bias baked into the data will make for a biased implementation of AI. The General Services Administration has its own bias toolkit that government at all levels can use to learn about sources of bias, understand the risks that certain marginalized groups face, and develop strategies for mitigating bias in their systems.
Implementing an AI system that prioritizes equity means using the principles of human-centered service design and delivery—so that along every step of the way, we’re thinking about the broader impact this change will have on people who need various government services. If the implementation of AI negatively impacts a certain community or demographic of people—or if it provides positive benefits disproportionately to some other group—it shouldn’t proceed until there are strategies in place to mitigate that. The metrics you’ve defined should also help you monitor any potential biases and inform your next steps.
Are there ways to ensure transparency and oversight about this application of AI and the use of data?
Using the data that AI collects can make sure that decisions are informed by evidence. And while it’s incredibly useful for informing product strategy, directing resources, and making process adjustments, that data must be managed carefully. Agencies should have clear, easy-to-understand policies for ethical data management when they’re considering implementing any new technology. In addition, agencies should also make sure they retain ownership of their data as they work with third party providers.
At Code for America, we think of data management at two distinct points: at source and downstream. At source data management means we’re thinking about the responsibilities associated with collecting people’s data. Our clients are trusting us with their personal information, and it’s our duty to safeguard it from the moment we receive it. Downstream data management means we’re guarding against potential unintended consequences from things like bias and breaches. Government teams can protect their clients by being rigorous about their data management and using analyses that reduce the risk of harm to communities who have been marginalized and harmed by automated technology in the past.
It sounds counterintuitive, but maintaining the human touch is critical to the success of any AI implementation.
What guardrails do we need to put in place to make sure this application of AI is safe?
It sounds counterintuitive, but maintaining the human touch is critical to the success of any AI implementation. It means humans are reviewing the decisions that AI is making based on the data we provide it, and humans are evaluating the benefits and costs of having AI perform those tasks.
One way in which AI can be made safer is to provide a fallback option so that a client can escalate their concerns and receive confirmation that someone will take action. When we designed a bot to respond to client queries during the SNAP application process, we made sure that we included a clear way for clients to contact our support staff.
How can we ensure an iterative and responsive approach to any problems, and how do we create feedback loops to get that information quickly?
Government teams should have the right people at the table from the beginning. This means knowing which departments need to be involved, from personnel management to IT, from innovation teams to caseworkers. When everyone is working collaboratively to design, implement, and measure a pilot AI project, it makes it easier to create feedback loops and identify places where you might need to pivot. Agile development frameworks, regular standup meetings, and shared data dashboards could be some of the methods that government uses to successfully put a new technology in place.
Putting it all together
AI might seem daunting, but it doesn’t have to be. The methods for ensuring success are the same that are at the core of any successful project: setting clear principles and strategies for collaboration, starting small and pivoting when necessary, and keeping people at the center of all decisions. There’s so much potential for AI to ease government workloads and improve people’s experience interacting with government services. By asking the right questions from the start, we can all bring that potential closer to reality.